Artificial Intelligence touches almost every aspect of our lives, from mobile banking and online shopping to social media and real-time traffic maps. But what happens when artificial intelligence is biased? What if it makes mistakes on important decisions — from who gets a job interview or a mortgage to who gets arrested and how much time they ultimately serve for a crime?
“These everyday decisions can greatly affect the trajectories of our lives and increasingly, they’re being made not by people, but by machines,” said UC Davis computer science professor Ian Davidson.
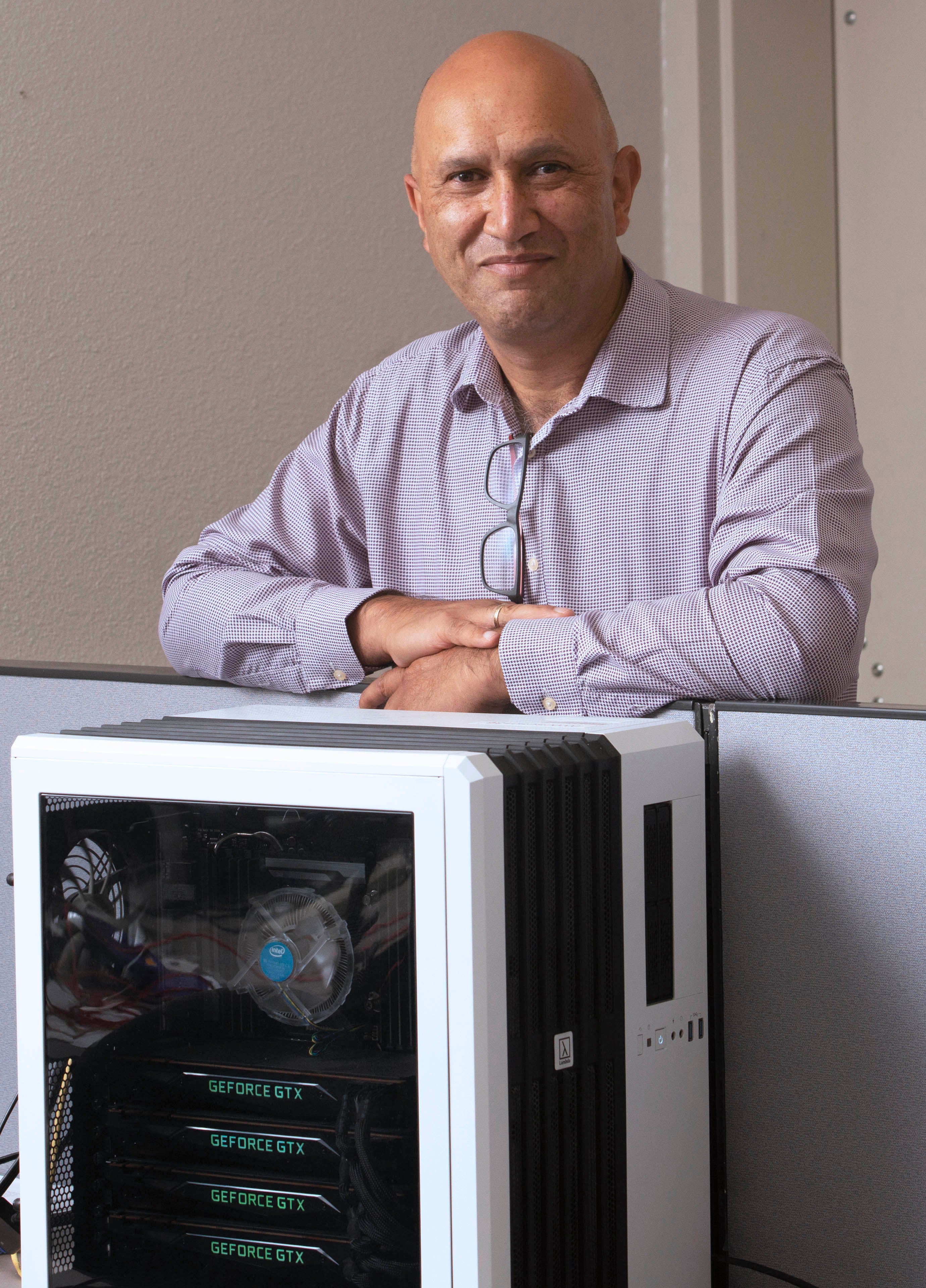
A growing body of research, including Davidson’s, indicates that bias in artificial intelligence can lead to biased outcomes, especially for minority populations and women.
Facial recognition technologies, for example, have come under increasing scrutiny because they’ve been shown to better detect white faces than they do the faces of people with darker skin. They also do a better job of detecting men’s faces than those of women. Mistakes in these systems have been implicated in a number of false arrests due to mistaken identity.
In fact, concern about bias in facial recognition technologies led to a number of bans on their use. In June 2019, San Francisco was among the first cities in the nation to ban the use of facial recognition technologies by the police and other city departments. The State of California followed suit in January 2020, imposing a three-year moratorium on the use of facial recognition technology in police body cameras.
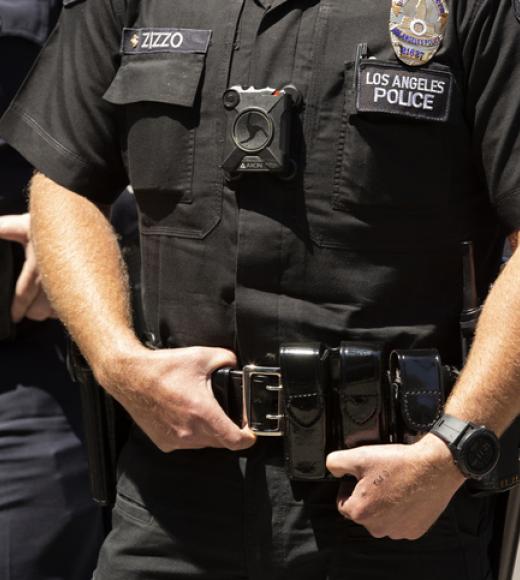
Has racial profiling gone digital?
A number of technologies take facial recognition a step further, analyzing and interpreting facial attributes and other data to make risk assessments, identify threats or unusual behavior. In the world of data surveillance, this is called “anomaly detection.” Increasingly, these technologies are deployed by law enforcement, airport security, and retail and event security firms.
In a recent study, Davidson and Ph.D. student Hongjing Zhang demonstrated that these types of anomaly detection algorithms are more likely to predict that African Americans or darker skinned males are anomalies.
“Since anomaly detection is often applied to people who are then suspected of unusual behavior, ensuring fairness becomes paramount,” Davidson said. “If one of these algorithms is used for surveillance purposes, it’s much more likely to identify people of color. If a white person walks in, it would not be likely to trigger an anomalous event. If a black person walks in, it would.”
“The machine is not biased. It has no moral compass. It’s just seen more white faces in the data it was trained on before and so it’s learned to associate that with normality.” — Ian Davidson, UC Davis computer science professor
That sounds a lot like computer-aided racial profiling.
“But it’s completely unintentional,” Davidson said. “The machine is not biased. It has no moral compass. It’s just seen more white faces in the data it was trained on before and so it’s learned to associate that with normality.”
Ensuring that AI is fair and free from bias is complex, he explained. His work shows that adding more people of color to the data the machine learns from helps, but it does not eliminate the issue.
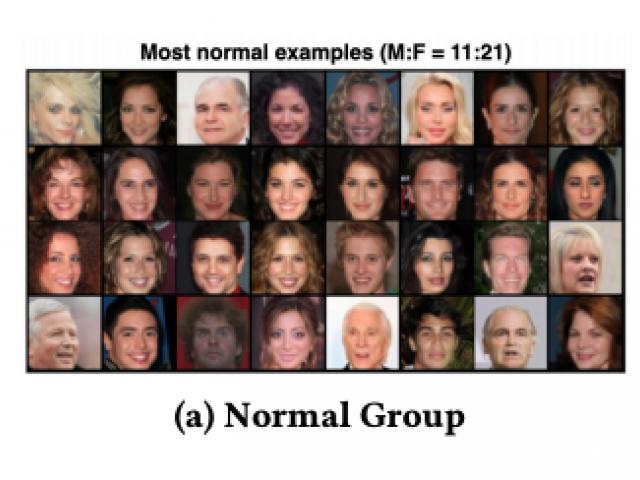
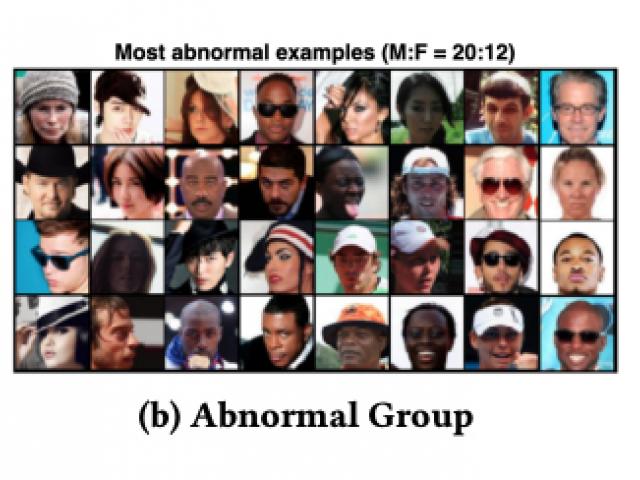
Bias reflects an unjust world
Examples abound of technologies that don’t perform accurately for people with darker skin. In February, the FDA warned that the pulse oximeters used to monitor oxygen saturation levels for COVID-19 patients may be less accurate for people with darker skin pigmentation. A study from Georgia Tech also found that people of color were more likely to be hit by driverless cars because object detection systems they use to recognize pedestrians don’t work as well on people with darker skin.
“Bias reflects a world that is unjust,” said Computer Science Professor Patrice Koehl. He teaches an ethics course that’s required for all UC Davis students majoring in computer science and engineering. Part of the course focuses specifically on bias in AI and other technologies.
“I want students to have an awareness of the problem and to understand why there are biases in our decisions,” Koehl said. “For example, if all your colleagues are white male, you’re unlikely to discuss problems associated with machine recognition of dark skin.”
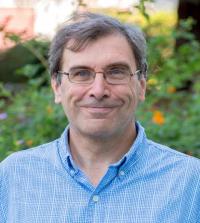
“The danger with bias comes from the fact that we consider AI as a system that can make decisions.” — Patrice Koehl, UC Davis computer science professor
This lack of diversity in the workforce has been a persistent problem in the technology sector. Nearly 80 percent of employees at Apple, Facebook, Google and Microsoft are male and there’s been little growth in Black, LatinX and Native representation since 2014, according to Mozilla’s 2020 Internet Health Report.
“The danger with bias comes from the fact that we consider AI as a system that can make decisions,” Koehl said. “You want that decision to be as informed as possible. If the information you provide is wrong or biased, the decision will be wrong.”
When it comes to developing future technologies, Koehl is optimistic that today’s students will do a better job. “The problem associated with AI was created in the last 20 years, partly by software engineers. If those engineers were able to create such a big problem, my hope is that the next generation of engineers will spend just as much time looking at the problems and identifying solutions,” he said.
Unraveling the tangled roots of bias
While there’s growing awareness of bias in artificial intelligence, there’s no simple solution. Bias can be introduced in a number of ways, beyond the software engineer developing a new technology. Artificial intelligence and machine learning algorithms rely on data, which is not always representative of minority populations and women. That’s because behind the data, the decisions — about which data to collect and how to use it — are still made by people.
“We cannot address bias and unfairness in AI without addressing the unfairness of the whole data pipeline system,” said Thomas Strohmer, director of UC Davis’ Center for Data Science and Artificial Intelligence Research, or CeDAR.
CeDAR is a hub for research activity focused on using AI for social good, from better healthcare to precision agriculture and combating climate change. Fighting bias and standing up for privacy is a natural part of that mission, Strohmer said.
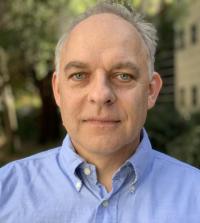
“Things like racial profiling existed before these tools. AI just enhances an existing bias." — Thomas Strohmer, director of CeDAR
“Things like racial profiling existed before these tools. AI just enhances an existing bias. If you feed a biased data set into an algorithm, the result will be a biased algorithm.”
Because new technologies are often adopted at scale, Strohmer noted, biases can quickly become widespread, and they’re not always easy to detect. To determine if there’s bias in a data set or an algorithm, you need access to the data and the algorithm.
Facial recognition, video analytics, anomaly detection and other kinds of pattern matching are being used in law enforcement — often out of public view.
AI in the shadows
Elizabeth Joh, a professor at the UC Davis School of Law, says this hidden nature of AI is a major concern.
“If there are problems in law enforcement, they are increasingly difficult for people to see,” said Joh, who has written extensively about technology, policing and bias. “Most people understand the utility of a firearm and a badge. If someone experiences excessive force by the police, we intuitively understand that. With technology, we might not even recognize that the problem exists. You might never know unless you become the target of that interaction.”
For this reason, she said accountability is crucial. She points to increasing experimentation with AI tools by police departments in towns and cities all across the country, all with little consideration for long-term consequences.
“We need to realize that these tools can quickly get out of hand or can be used in ways that are unexpected and have socially harmful consequences or disparate consequences,” Joh said. “A certain amount of bias has always existed in policing. Hidden technologies can exacerbate the problem immensely.”
Joh added it’s not too late for police departments and other organizations to take a step back and ask the most fundamental questions about the use of AI: Should we be using these tools at all?
Harnessing AI’s power for good
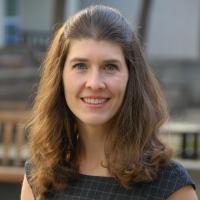
“Education, training and promoting diversity are key to addressing how technology is perpetuating bias,” said Pamela Reynolds, associate director of the UC Davis DataLab: Data Science and Informatics department. “Just as AI is contributing to these persistent societal problems, it can also be empowering for uncovering bias and finding solutions.”
In this regard, the DataLab leads by example. It supports a diverse faculty and affiliates program and hosts events for women and underrepresented individuals in data science.
WHAT DO DATING TECHNOLOGY AND ALZHEIMER’S HAVE IN COMMON?
UC Davis neuropathologist Brittany Dugger, along with researchers at UC San Francisco, has found a way to teach a computer to precisely detect one of the hallmarks of Alzheimer’s disease in human brain tissue, delivering a proof of concept for a machine-learning approach capable of automating a key component of Alzheimer’s research. Read the research, and watch the video.
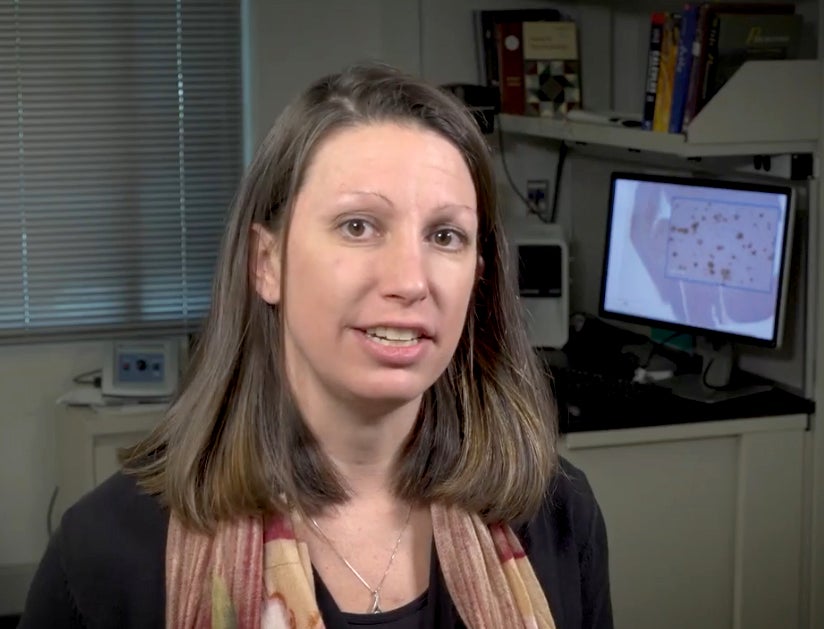
“In data science, we are working with large sets of information and these inevitably reflect the structural inequalities of our society,” said Reynolds, who is an experimental ecologist by training. “Without a critical and inclusive approach to data and the AI tools it enables, we run the risk of reproducing past injustices.”
The DataLab helps students and researchers understand the complexity of large data systems and how technologies and computational methods work. This has added value to a number of research projects, including a three-year study to improve early diagnosis of Alzheimer’s in women and communities of color, being conducted by Brittany Dugger, assistant professor of pathology and laboratory medicine, and her team at UC Davis Health.
Trust will require transparency
The trend toward greater use of artificial intelligence shows no signs of abating — whether it’s to improve healthcare, recommend movies via a streaming service, conduct surveillance, or any of a myriad of other uses.
For that reason, transparency in AI is more important than ever. According to Davidson, that will require fairness, explainability and privacy.
“As machines replace humans and make more decisions, we need to be able to trust them,” he said. “That includes understanding exactly how machine algorithms are processing information and how decisions are being made.”
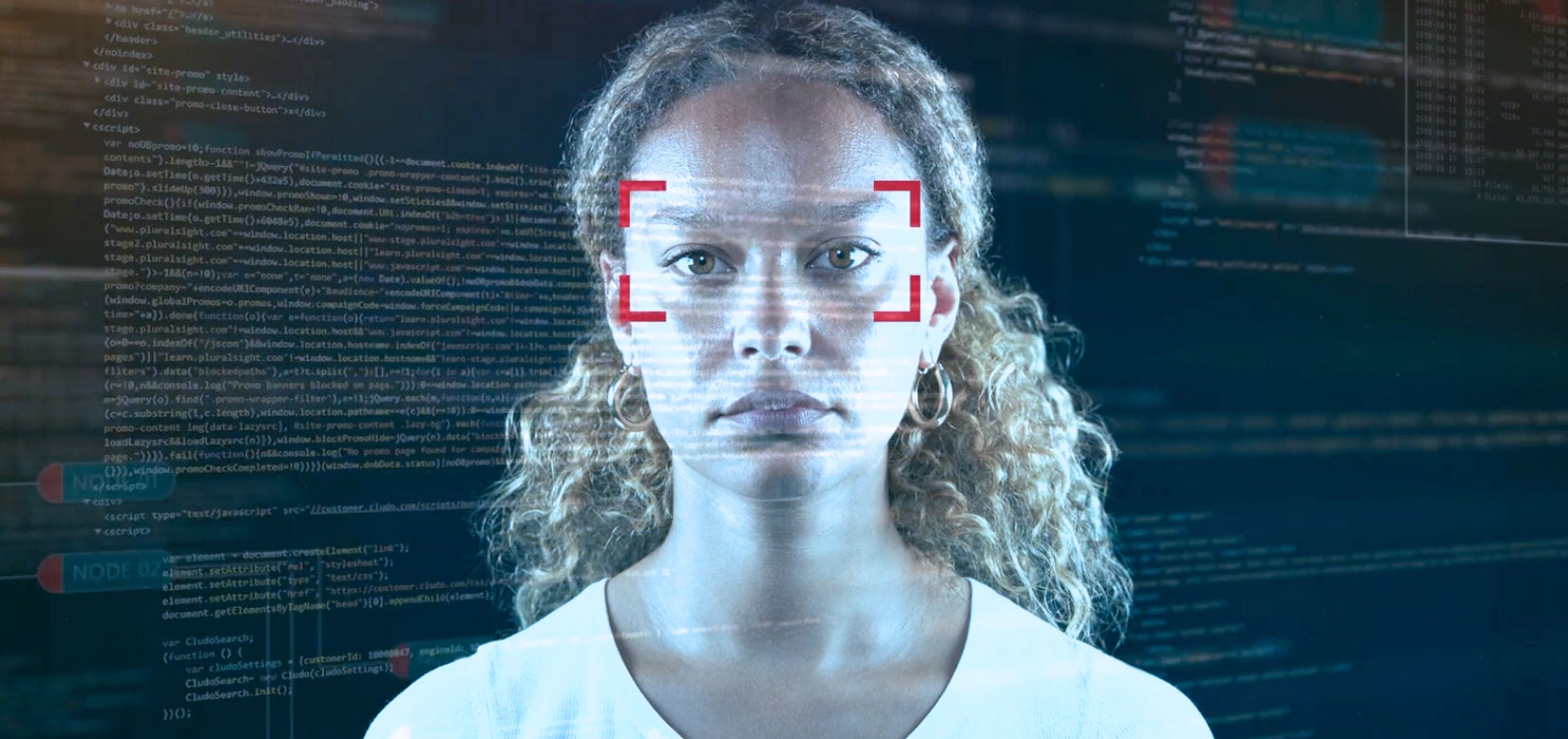
Related Stories
Facial Recognition Technology and Racial Bias
UC Davis Law Professor was a panelist for the online webinar “Facial Recognition Technology and its Algorithmic Racial Bias.” Presented in September 2020 by the University of Minnesota Law School, the webinar explored some of the implications of the growing use of video and AI technology in policing and the justice system and its relation to efforts to eliminate bias.
Media Resources
Catherine Kenny, UC Davis News and Media Relations, 530-752-3140, cmkenny@ucdavis.edu